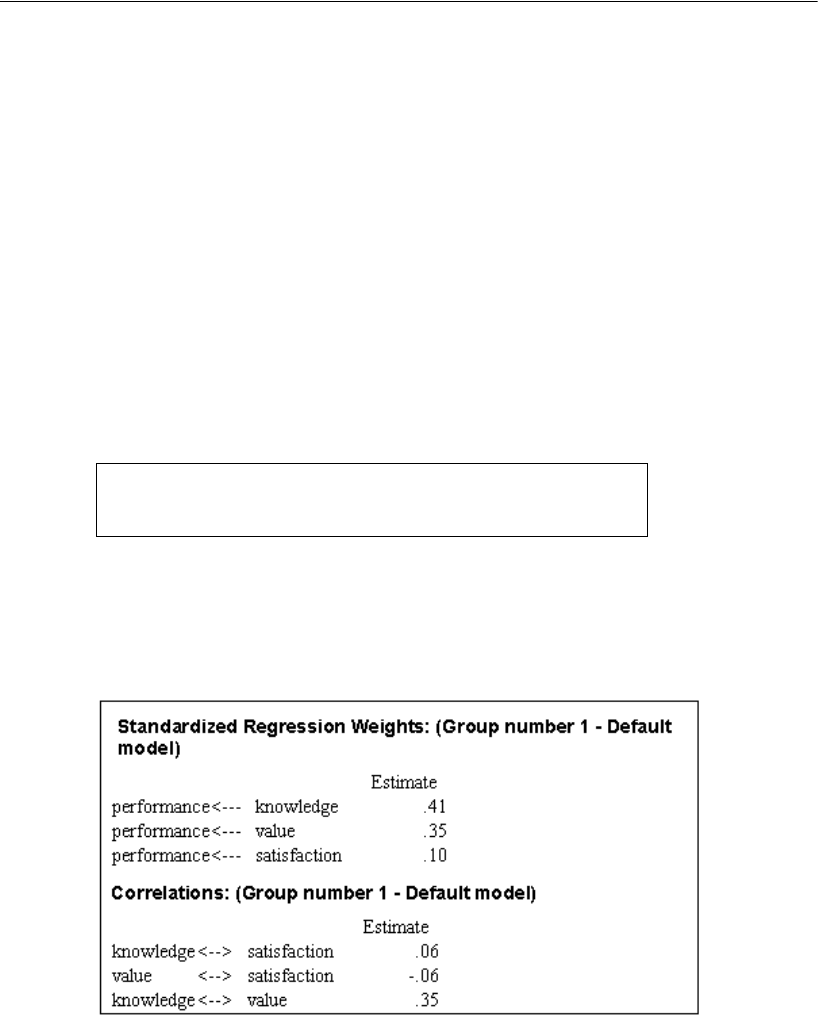
73
Conventional Linear Regression
of the same factor. Extending this, the product of the squared regression weight and the
error variance is always a constant. This is what we mean when we say the regression
weight (together with the error variance) is unidentified. If you assign a value to one
of them, the other can be estimated, but they cannot both be estimated at the same time.
The identifiability problem just discussed arises from the fact that the variance of a
variable, and any regression weights associated with it, depends on the units in which
the variable is measured. Since error is an unobserved variable, there is no natural way
to specify a measurement unit for it. Assigning an arbitrary value to a regression weight
associated with error can be thought of as a way of indirectly choosing a unit of
measurement for error. Every unobserved variable presents this identifiability
problem, which must be resolved by imposing some constraint that determines its unit
of measurement.
Changing the scale unit of the unobserved error variable does not change the overall
model fit. In all the analyses, you get:
There are four sample variances and six sample covariances, for a total of 10 sample
moments. There are three regression paths, four model variances, and three model
covariances, for a total of 10 parameters that must be estimated. Hence, the model has
zero degrees of freedom. Such a model is often called saturated or just-identified.
The standardized coefficient estimates are as follows:
Chi-square = 0.00
Degrees of freedom = 0
Probability level cannot be computed